How to Use Open Data to Predict Forest Fires π₯
Proactive human intervention is necessary to mitigate these effects and prevent ongoing carbon loss. π
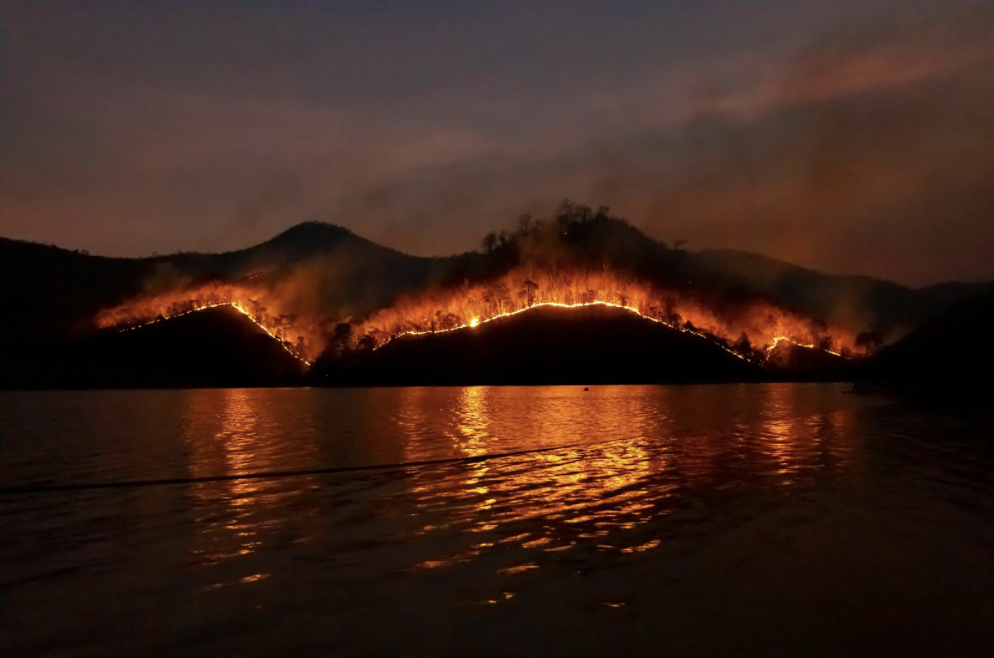
π Overview
In 2023, the world witnessed devastating impacts due to wildfires, with record numbers of forests lost across various continents. These fires were driven by a combination of factors, including climate change, which has led to drier and hotter conditions conducive to wildfires.
But what if we could use tree data to predict where human intervention could prevent mass fires from happening in the first place? It turns out that we might be able to. π²
This article by Peeler et al. discusses identifying areas in western US conifer forests where proactive forest management can significantly reduce wildfire-caused carbon loss. It highlights the need for tried-and-tested techniques, such as forest thinning and prescribed burning, especially in California, New Mexico, and Arizona, which are highly vulnerable. Most crucially, it suggests that we can use data models to predict where such techniques will be most effective. The authors emphasize the importance of collaborative and equitable management strategies to mitigate climate and wildfire risks while maintaining carbon sequestration and supporting community resilience against such disasters. π
π² The Importance of Intervention
High-severity wildfires in frequent-fire forests disrupt carbon recovery by destroying mature trees, which impedes the regeneration of forests and thus their ability to sequester carbon again.
Why it matters: Such wildfires can create a cycle of more frequent and intense fires, further reducing carbon stocks. Climate change exacerbates this issue by creating hotter, drier conditions that hinder tree regeneration even further. Proactive human intervention is necessary to mitigate these effects and prevent ongoing carbon loss. π
How do you think targeted interventions could help in breaking the cycle of frequent wildfires?
π Studying Current Developments
The study developed a geospatial database that integrates factors like annual burn probability, total carbon, potential carbon loss, and carbon recovery post-wildfire. These indicators were normalized and weighted to identify areas most at risk from wildfires, aiming to inform targeted forest management practices for reducing wildfire-caused carbon loss. π
Key insight: This approach helps in pinpointing regions where interventions like thinning and prescribed burns could be most effective in preventing catastrophic wildfires and preserving carbon stocks.
What other factors do you think should be considered in wildfire risk assessments?
π Strategies for Mitigation Using Data
The article advocates for using open environmental data to enhance the accuracy and effectiveness of forest management.
Why it matters: By refining risk assessments and management approaches with precise data, targeted interventions can be more effectively applied to specific forest conditions. The ultimate goal is to develop online tools that could inform and improve forest management practices to prevent wildfires, relying on contributions from local communities to enrich the data. π²
How can local community input enhance the effectiveness of these data-driven tools?
π§ Technical Insights
Peeler et al. utilized bivariate maps (maps of two or more variables) and categorized data into quantiles for carbon exposure, sensitivity, and vulnerability to wildfires.
How it works: This method provides a clear framework for identifying areas at different levels of wildfire risk by evaluating the potential for carbon loss and the capacity for carbon recovery. This nuanced assessment helps in prioritizing regions where carbon stocks are most at risk from wildfires. π₯
What role do you think advanced mapping techniques could play in other areas of environmental management?
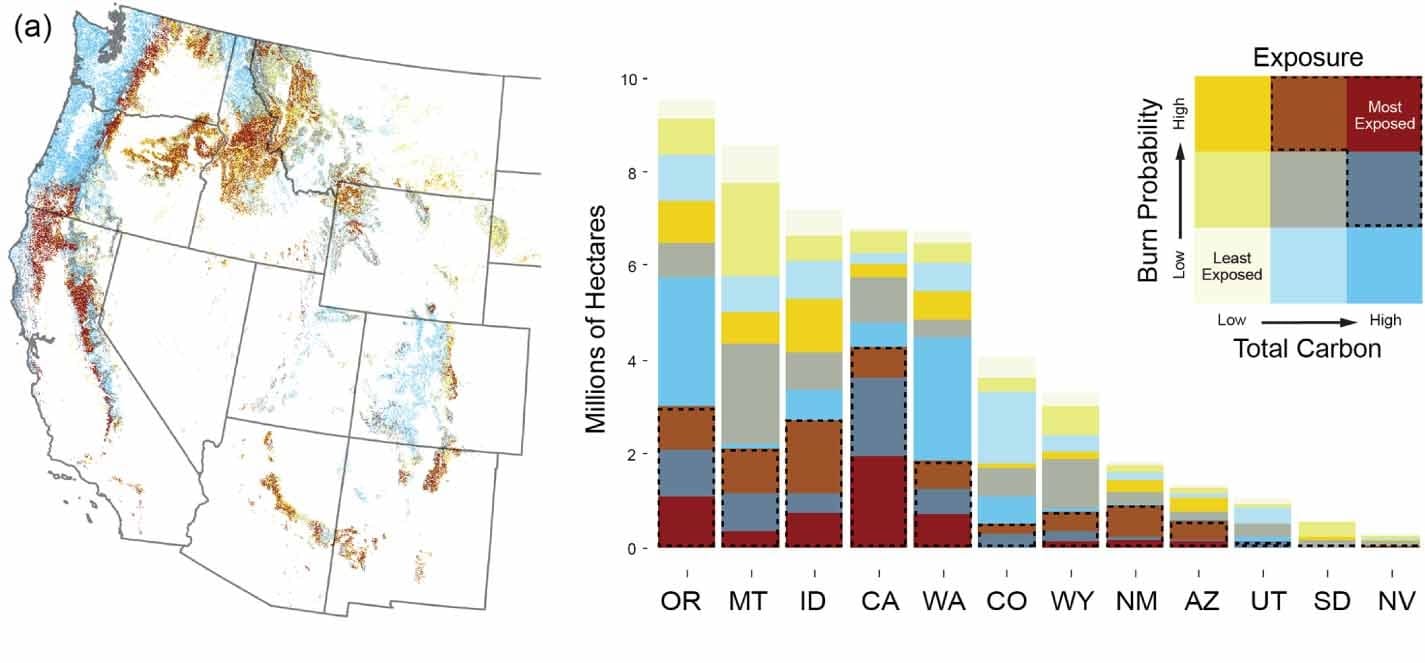
π Data Accessibility and Tools
Peeler, Jamie et al. (2023). Geospatial data from: Identifying opportunity hot spots for reducing the risk of wildfire-caused carbon loss in western US conifer forests [Dataset]. Dryad. Access the data here.
Why it matters: The data can be opened using R or ArcGIS Pro, providing valuable resources for researchers and forest managers to develop and implement effective wildfire prevention strategies. π
Curious to explore the data? Dive into the resources and see how you can contribute to improving wildfire management.