AI in the Mangroves: Charting Asir’s Path to Coastal Revival
A Generalized Additive Model (GAM) pinpoints areas ideal for mangrove restoration with 91% predictive accuracy.
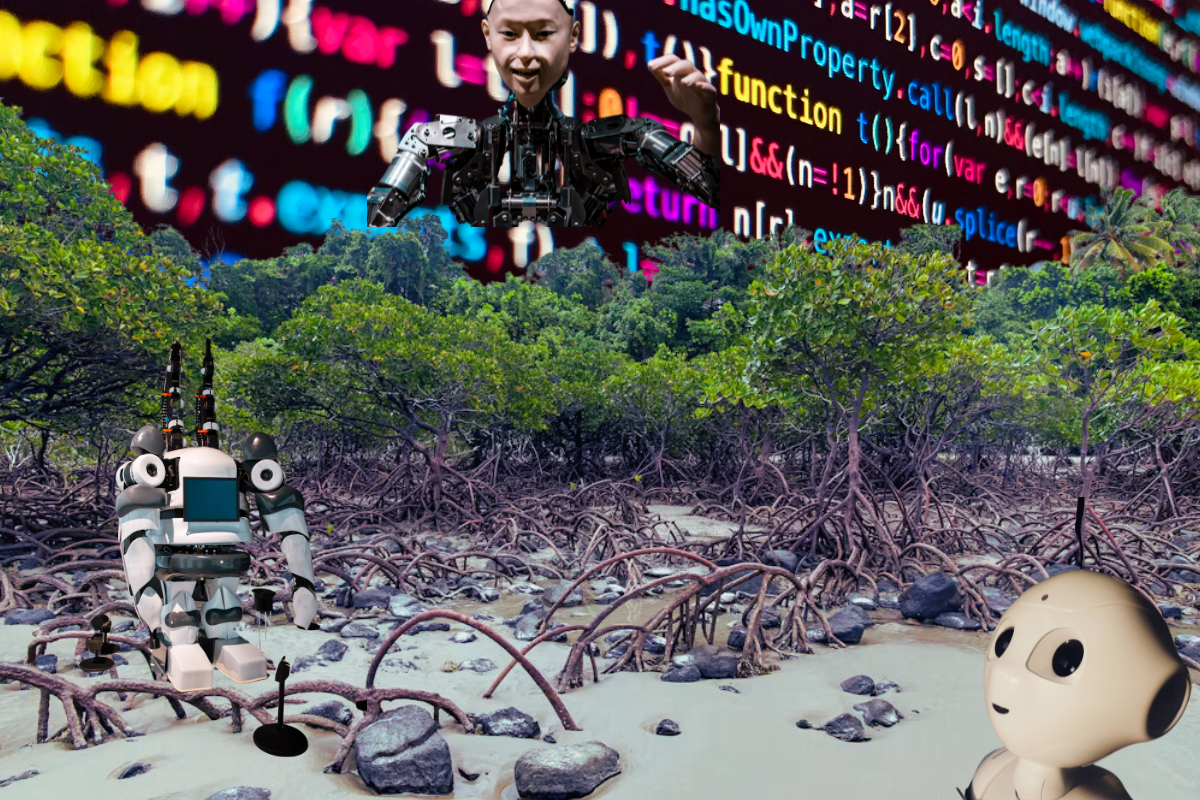
An Algorithm that Does Something Useful
Tucked along Saudi Arabia’s stunning Red Sea coast, the mangroves of Asir are essentially nature’s bouncers, keeping storm surges, erosion, and biodiversity loss at bay. But even the toughest guardians have their limits. Climate change is turning up the heat—literally—and these vital ecosystems are feeling the burn. Mangroves also have to contend with threats from new road construction, coastal development, and tidal variations.
Cue the unlikely hero of our story (or at least, one with great potential): machine learning (ML), swooping in like the nerdy earth-Avenger nobody expected, ready to map, analyze, and potentially save the day. A new study by Al Huqail et al. (2024) in MDPI delves into this.
Would you believe if I told you Camels are acrossing Asir’s coast, well here’s a video a magical one 😍 #Camels in #Asir go long distances in search of food in the #mangroves, travelling at least 3 kilometers to eat. #SaudiArabia #mothernature
— The Jeddah Times (@thejeddahtimes) November 19, 2019
#traveling #explore #asir pic.twitter.com/uY5AzSaatp
The mangroves of Asir.
Where Data Meets Nature: Mangrove Mapping with Landsat
To evaluate the mangroves' condition, researchers analyzed three decades of Landsat satellite imagery (1991–2023), applying machine learning to identify patterns in environmental health. Key metrics included:
- Normalized Difference Water Index (NDWI): A measure of water availability. The study found no significant change over three decades, underscoring the need for improved hydrological systems to support future reforestation efforts.
- Normalized Difference Vegetation Index (NDVI): An indicator of vegetation health showing that Asir's mangroves retain vitality despite challenges.
- Land Surface Temperature (LST): Correlating temperature fluctuations with ecosystem stress.
The real game-changer? A Generalized Additive Model (GAM) was employed to synthesize these variables into a detailed suitability map, pinpointing areas ideal for reforestation with 91% predictive accuracy, transforming raw data into actionable insights.
It should be noted however that the study emphasizes that the satellite data will need to be validated (dare we even say - "ground truthed"?) with on-the-ground surveys.
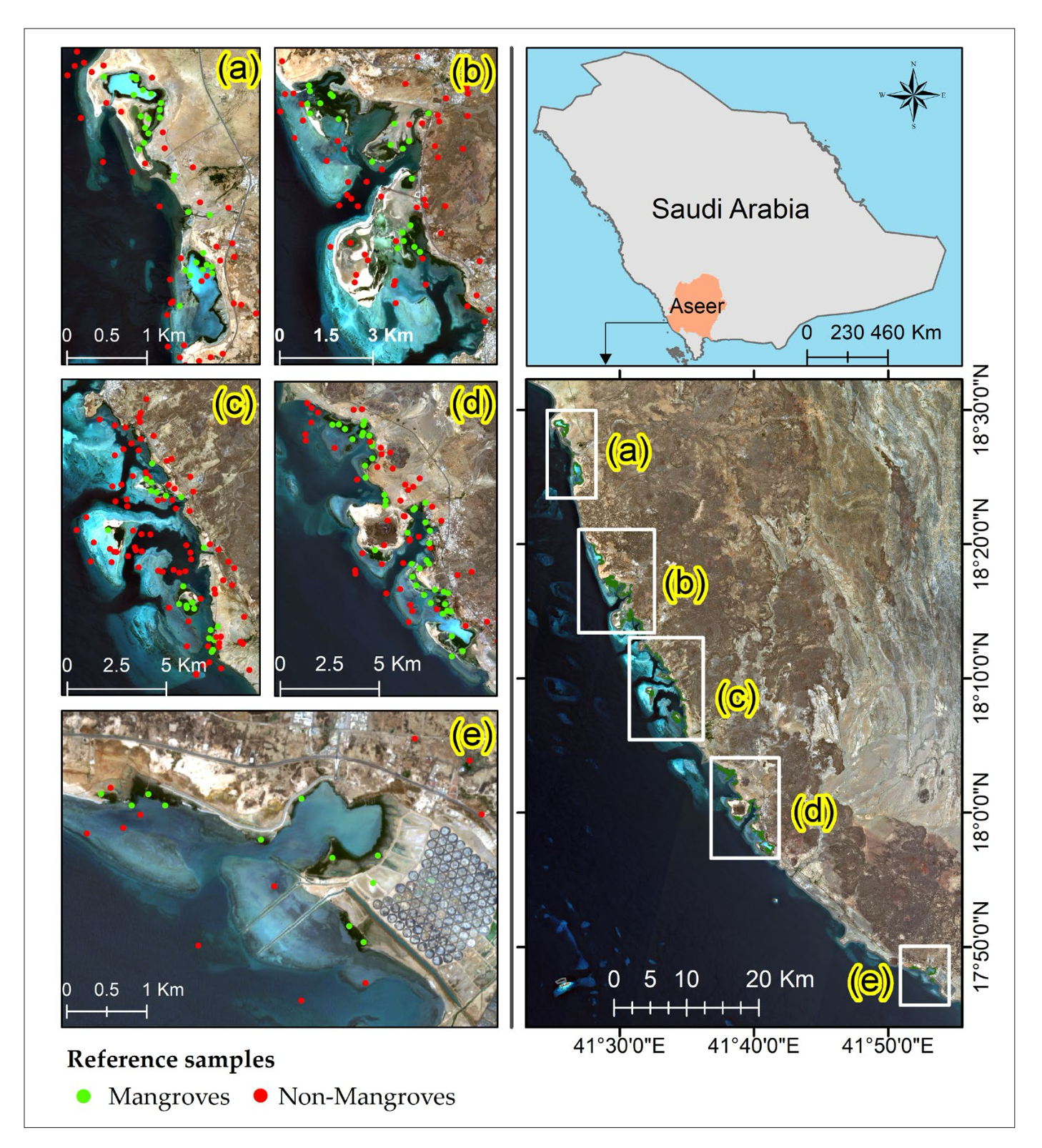
How Targeted Reforestation Can Support Vision 2030
Using GAM's predictive capabilities, the researchers identified Group E, an area with 77% suitability for mangrove replanting. But challenges persist—particularly water scarcity. The NDWI findings underscore the need to address hydrological systems to sustain new plantings.
Recommended Actions:
- Restoring Hydrological Systems:
Address water scarcity by revitalizing tidal and hydrological flows. The study’s NDWI analysis shows that stagnant water conditions are a major limiting factor for mangrove growth, making hydrological improvements critical to reforestation success. - Focusing on High-Suitability Zones:
Use the Generalized Additive Model (GAM) to guide restoration efforts. Priority zones like Group E, identified as 77% suitable for replanting, offer the best chances of success. Targeting these areas ensures efficient use of resources and maximizes ecological impact. - Engaging Local Communities:
Involve local stakeholders in conservation and monitoring efforts. Community participation is vital for long-term sustainability, empowering residents to protect mangroves and contribute to restoration projects. - Leveraging Long-Term Data:
Base conservation strategies on trends revealed by decades of satellite imagery. By analyzing NDWI, NDVI, and LST metrics, the study emphasizes the importance of adapting restoration efforts to evolving ecological conditions. - Utilizing GAM for Decision-Making:
Incorporate advanced modeling tools like GAM into mangrove management plans. GAM’s high predictive accuracy (91%) provides a reliable foundation for identifying suitable reforestation areas and optimizing conservation outcomes.
These strategies not only support Saudi Arabia’s Vision 2030 ecological goals but also provide a replicable model for global restoration initiatives.
Democratizing Mangrove Restoration Through Open Data
By publishing their machine learning models, GAM framework, and datasets, they’ve provided tools that others can use, adapt, and improve—turning a local effort into a global resource.
Here’s why this matters for forestry:
-Replication and Scaling: Mangrove ecosystems aren’t the only forests under threat. By making their framework accessible, researchers worldwide can adapt it for other forest types—tropical rainforests, peatlands, or coastal wetlands—facing similar challenges.
-Data-Driven Restoration: Forestry enthusiasts, in their quest to plant one trillion trees globally, have struggled to prioritize areas for replanting—and it is crucial to prioritize the right tree in the right place. This methodology offers a clear, evidence-backed way to identify high-priority zones, saving time and resources.
-Global Collaboration: Shared tools mean shared progress. Open data lets conservation teams across the globe pool knowledge, compare results, and accelerate innovations in restoration techniques.
This isn’t just a win for mangroves—it’s a blueprint for smarter, faster forestry restoration everywhere. Sharing these methodologies ensures that efforts to combat deforestation and climate change are more effective, coordinated, and scalable.
Final Notes on Huqail et al.
Mangroves might not have the star power of pandas or the Instagram aesthetic of coral reefs, but they’re punching way above their weight in ecological importance. Thanks to a little ML magic, Asir’s mangroves might just get the glow-up they deserve.
The success of AI-driven restoration in Asir highlights the potential for similar methodologies worldwide, paving the way for smarter, data-driven approaches to reforestation and climate resilience. So here’s to a future where (hopefully) algorithms do less “suggesting new sweatpants” and more “saving the planet.”
Sources
Al-Huqail, A.A.; Islam, Z.; Al-Harbi, H.F. An ML-Based Ensemble Approach for the Precision Classification of Mangroves, Trend Analysis, and Priority Reforestation Areas in Asir, Saudi Arabia. Sustainability 2024, 16, 10355. https://doi.org/10.3390/su162310355. Retrieved from https://www.mdpi.com/2071-1050/16/23/10355.
World Economic Forum. One Trillion Trees: World Economic Forum launches plan to help nature and the climate. (2020, January 22). Retrieved from https://www.weforum.org/stories/2020/01/one-trillion-trees-world-economic-forum-launches-plan-to-help-nature-and-the-climate/.
Perry, Nick. (2024, March 30). Planting the wrong trees could do more harm than good for the planet. Phys.org. Retrieved from https://phys.org/news/2024-03-trees-wrong-planet.html.
Vision 2030. Retrieved from https://vision2030.gov.sa/en.
Images courtesy of Ali Shah Lakhani, Maximal Focus, Possessed Photography, and David Clode.
Edited by Chris Harris
This work is licensed under a
Creative Commons Attribution 4.0 International License.